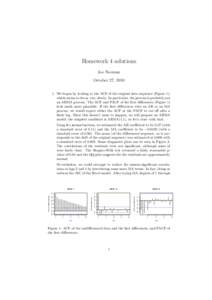 Date: 2010-11-23 19:26:03Time series models Noise Time series analysis Covariance and correlation Moving-average model Partial autocorrelation function Autoregressive integrated moving average Akaike information criterion QQ plot BoxJenkins | | Homework 4 solutions Joe Neeman October 27, We began by looking at the ACF of the original data sequence (Figure 1), which seems to decay very slowly. In particular, the process is probably not an ARMA process. TAdd to Reading ListSource URL: www.stat.berkeley.eduDownload Document from Source Website File Size: 115,89 KBShare Document on Facebook
|