111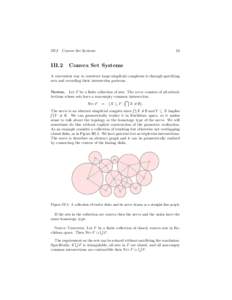 | Add to Reading ListSource URL: www.cs.duke.edu- Date: 2006-10-16 09:23:18
|
---|
112 | Add to Reading ListSource URL: materials.dagstuhl.de- Date: 2015-09-02 14:11:33
|
---|
113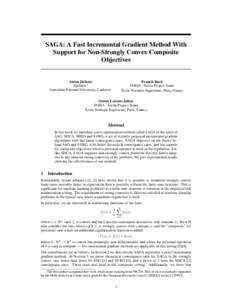 | Add to Reading ListSource URL: papers.nips.cc- Date: 2014-12-02 18:40:41
|
---|
114![Hit-and-Run for Sampling and Planning in Non-Convex Spaces arXiv:1610.08865v1 [stat.CO] 19 Oct 2016 Yasin Abbasi-Yadkori Queensland University of Technology Hit-and-Run for Sampling and Planning in Non-Convex Spaces arXiv:1610.08865v1 [stat.CO] 19 Oct 2016 Yasin Abbasi-Yadkori Queensland University of Technology](https://www.pdfsearch.io/img/1812c8f18b474749297ae900d6cf2d98.jpg) | Add to Reading ListSource URL: arxiv.org- Date: 2016-10-27 20:13:57
|
---|
115 | Add to Reading ListSource URL: www.alexschied.de- Date: 2009-03-07 10:48:57
|
---|
116 | Add to Reading ListSource URL: jmlr.org- Date: 2014-05-28 14:26:58
|
---|
117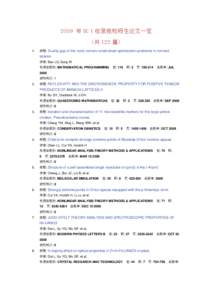 | Add to Reading ListSource URL: lib.hrbust.edu.cn- Date: 2010-03-15 22:08:03
|
---|
118 | Add to Reading ListSource URL: www.mat.univie.ac.at- Date: 2015-07-13 19:52:37
|
---|
119 | Add to Reading ListSource URL: www.math.purdue.edu- Date: 2005-08-03 16:12:42
|
---|
120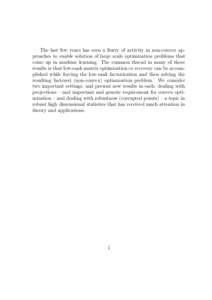 | Add to Reading ListSource URL: mmds-data.org- Date: 2016-06-23 15:50:48
|
---|