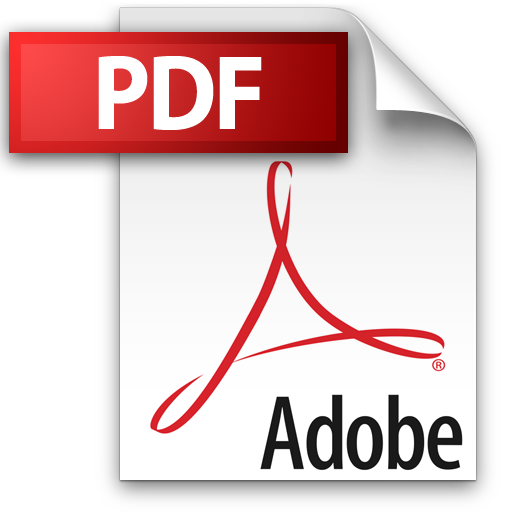 Date: 2018-02-21 18:52:27Ambient intelligence Internet of things Long short-term memory Scheduling Future Technology Applied mathematics | | Cellular Network Traffic Scheduling using Deep Reinforcement Learning Sandeep Chinchali, et. al. Marco Pavone, Sachin Katti Stanford University AAAI 2018Add to Reading ListSource URL: platformlab.stanford.eduDownload Document from Source Website File Size: 3,46 MBShare Document on Facebook
|