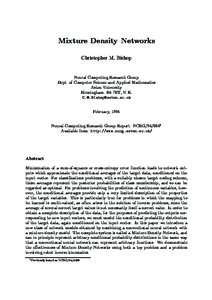 Date: 2017-02-05 19:13:20Mathematical analysis Probability and statistics Probability theory Machine learning Computational neuroscience Computational statistics Artificial neural networks Bayesian statistics Gaussian function Mixture model Mixture distribution Joint probability distribution | | Mixture Density Networks Christopher M. Bishop Neural Computing Research Group Dept. of Computer Science and Applied Mathematics Aston UniversityAdd to Reading ListSource URL: publications.aston.ac.ukDownload Document from Source Website File Size: 428,26 KBShare Document on Facebook
|