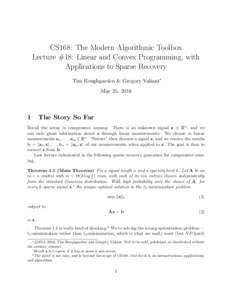 Date: 2016-06-04 09:49:43Mathematical optimization Numerical analysis Mathematical analysis Operations research Linear programming Convex optimization Convex analysis Ellipsoid method Feasible region Convex function Linear inequality Candidate solution | | CS168: The Modern Algorithmic Toolbox Lecture #18: Linear and Convex Programming, with Applications to Sparse Recovery Tim Roughgarden & Gregory Valiant∗ May 25, 2016Add to Reading ListSource URL: theory.stanford.eduDownload Document from Source Website File Size: 305,83 KBShare Document on Facebook
|