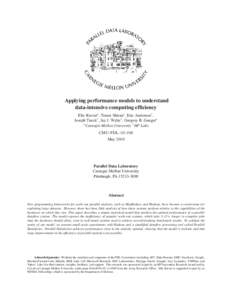
| Document Date: 2010-10-14 00:07:50 Open Document File Size: 303,89 KBShare Result on Facebook
Company IBM / APC / Microsoft Research / NEC Laboratories / Sun / NetApp / LSI / HP / Oracle / Yahoo! Labs / Data Domain / Symantec / Seagate / Hewlett-Packard Labs / EMC / Google / VMWare / Intel / Facebook / Hitachi / / Currency USD / / Facility Carnegie Mellon University / Parallel Data Laboratory Carnegie Mellon University / / IndustryTerm dataflow systems / map-reduce systems / map-reduce computing / parallel applications / software stack / simplified dataflow processing tool / hypothetical systems / scale-out systems / online transaction records / file systems / data-intensive computing / similar data processing tasks / computing / cross-product / cloud computing / map-reduce style systems / parallel systems / data-intensive computing systems / well-tuned systems / data-intensive computing efficiency / / Organization Army Research Office / Carnegie Mellon University / Department of Defense / Parallel Data Laboratory Carnegie Mellon University Pittsburgh / / Person Gregory R. Ganger / Dw N Dw / Joseph Tucek / Eric Anderson / Elie Krevat / Tomer Shiran / Jay J. Wylie / Dw N Table / / Position Model / model for both production and hypothetical systems / representative / Dean / / ProvinceOrState Manitoba / Pennsylvania / / PublishedMedium computing Today / / Technology load balancing / simulation / operating system / DBMS / /
SocialTag |