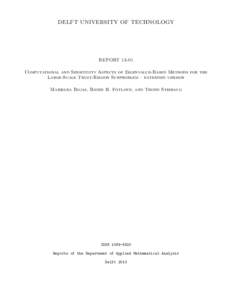 Date: 2013-04-17 08:35:19Numerical linear algebra Matrix theory Abstract algebra Singular value decomposition Eigenvalues and eigenvectors Eigenvalue algorithm Lanczos algorithm Inverse problem Preconditioner Algebra Linear algebra Mathematics | | DELFT UNIVERSITY OF TECHNOLOGY REPORTComputational and Sensitivity Aspects of Eigenvalue-Based Methods for the Large-Scale Trust-Region Subproblem – extended version Marielba Rojas, Bjørn H. Fotland, and TrondAdd to Reading ListSource URL: www.ewi.tudelft.nlDownload Document from Source Website File Size: 686,44 KBShare Document on Facebook
|