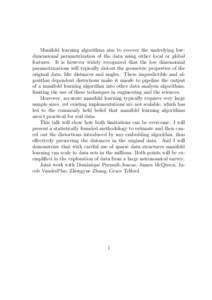 Date: 2016-06-23 15:50:48Machine learning Cybernetics Learning Manifold Cognition Dimension reduction Multivariate statistics Cognitive science Nonlinear dimensionality reduction | | Manifold learning algorithms aim to recover the underlying lowdimensional parametrization of the data using either local or global features. It is however widely recognized that the low dimensional parametrizations will Add to Reading ListSource URL: mmds-data.orgDownload Document from Source Website File Size: 11,77 KBShare Document on Facebook
|