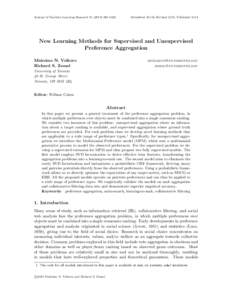 Date: 2014-03-31 14:49:59Psychometrics Choice modelling Preference Model theory Aggregation problem Economics Microeconomics Pairwise comparison | | Journal of Machine Learning Research1022 Submitted 10/12; Revised 9/13; Published 3/14 New Learning Methods for Supervised and Unsupervised Preference AggregationAdd to Reading ListSource URL: www.cs.toronto.eduDownload Document from Source Website File Size: 1,43 MBShare Document on Facebook
|