21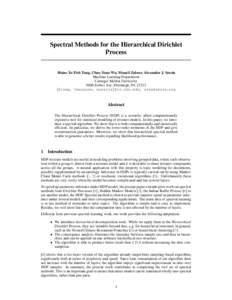 | Add to Reading ListSource URL: manzil.mlLanguage: English - Date: 2016-01-03 02:26:58
|
---|
22 | Add to Reading ListSource URL: alex.smola.orgLanguage: English - Date: 2013-09-09 02:28:41
|
---|
23 | Add to Reading ListSource URL: alex.smola.orgLanguage: English - Date: 2013-09-09 02:28:42
|
---|
24 | Add to Reading ListSource URL: alex.smola.orgLanguage: English - Date: 2013-09-09 02:28:40
|
---|
25 | Add to Reading ListSource URL: alex.smola.orgLanguage: English - Date: 2015-03-18 13:00:13
|
---|
26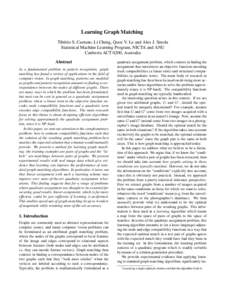 | Add to Reading ListSource URL: tiberiocaetano.comLanguage: English - Date: 2008-05-10 06:37:14
|
---|
27 | Add to Reading ListSource URL: alex.smola.orgLanguage: English - Date: 2015-04-30 21:00:10
|
---|
28 | Add to Reading ListSource URL: suvrit.deLanguage: English - Date: 2016-03-19 19:42:54
|
---|
29 | Add to Reading ListSource URL: alex.smola.orgLanguage: English - Date: 2013-09-09 02:28:38
|
---|
30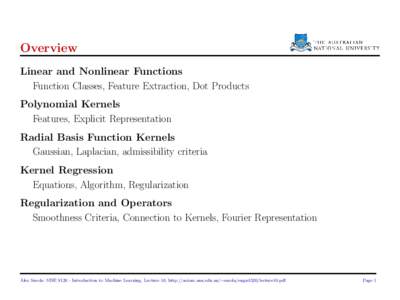 | Add to Reading ListSource URL: alex.smola.orgLanguage: English - Date: 2013-09-09 02:28:41
|
---|